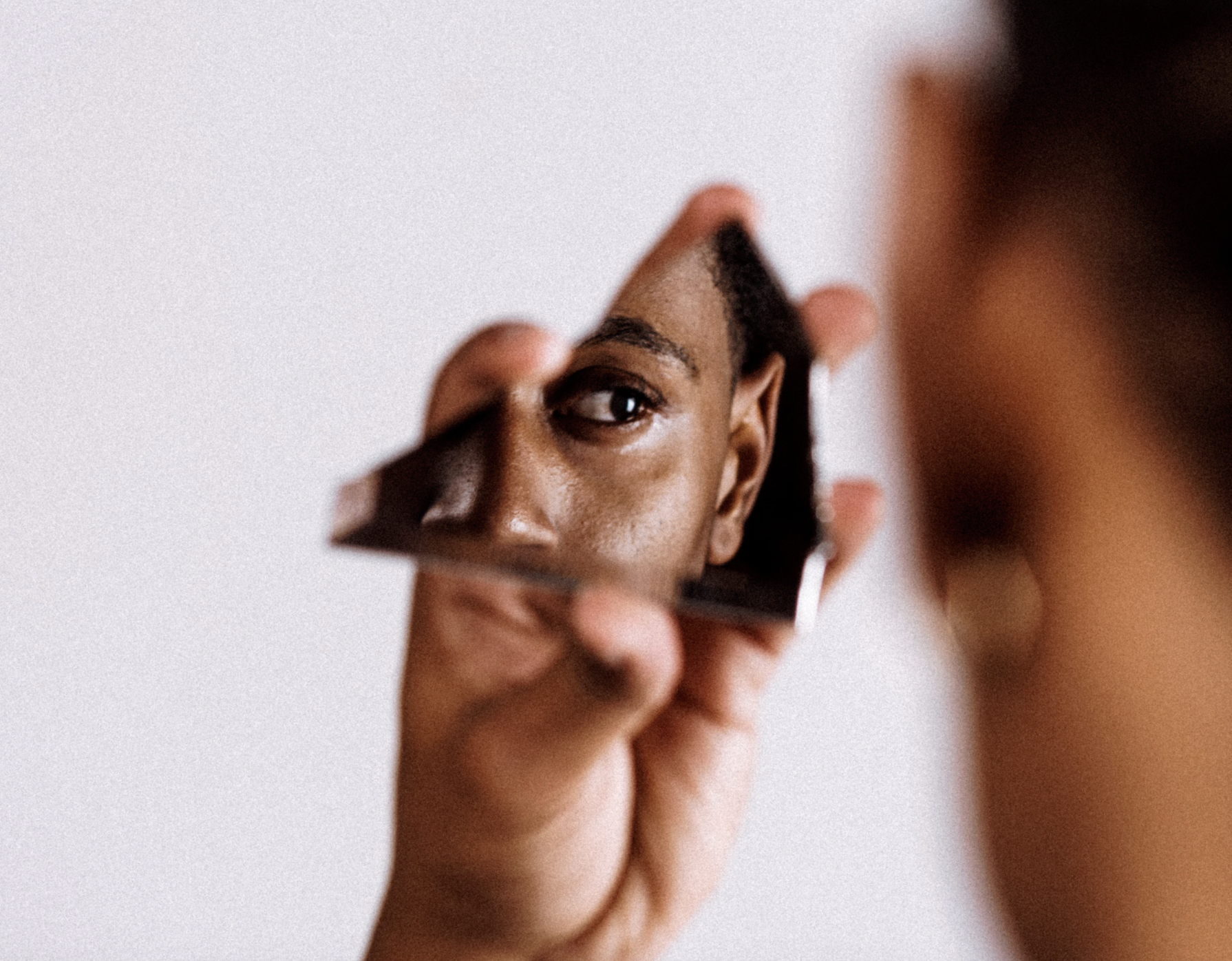
In 2018, research reports from the ACLU emerged indicating that Amazon’s facial recognition technology had reportedly “confused” the faces of 28 congressmen with the faces of known criminals. Let’s unpack that statement. First, Amazon has been developing facial recognition technology, called Rekognition, which can provide “highly accurate facial analysis and facial recognition on images and video.” In 2018, Amazon was actively making movements towards selling this technological tool to law enforcement. The ACLU, among other organizations and individuals, were concerned with the implications of utilizing such technology. In turn, the ACLU conducted its own study employing Rekognition facial technology. Their research uncovered that Rekognition technology was not as accurate of a facial recognition tool as had been perceived, and resulted in a large number of mismatched faces. Most importantly, the ACLU reported that Rekognition disproportionately misidentified faces of people of color- as highlighted by the 28 faces of congressmen that were misidentified as being the faces of known criminals. While Amazon and the ACLU debated over these results, the findings published by the ACLU point towards a bigger issue- in what ways are racial biases manifesting themselves in facial recognition technology and how can this cause harm to communities of color?
How Racial Biases Became a part of Facial Recognition Technology
For starters, let’s begin by discussing how racial biases even become a part of facial recognition technology. Typically, when people think of automated technology systems, they think of them as being scientific, neutral, and free of human prejudices. But as uncovered through the ACLU, and other similar studies, is that facial recognition technology is not free from the inclusion of racial biases. In fact, it appears that our technologies are inundated with biases and discrimination, being reflective of the same biases and discrimination that are currently present within our society. As these technologies begin to reflect this, it in turns acts as a medium that reinforces these biases at a larger scale. And facial recognition technology is doing just that. In addition to the study conducted by the ACLU, research conducted by MIT also found that facial recognition technology was more likely to misidentify the faces of women, as well as people of color (and especially women of color). The potential consequences of the misidentification of people of color in a technology that has the potential to be utilized by law enforcement is huge. In the United States, Black people are up to 2.5 times more likely to be targeted by police surveillance, and as a result, are currently overrepresented in mug-shot data bases across the country. So not only are Black people, and other communities of color, more likely to be misidentified by facial recognition technology, but if employed by law enforcement, they are also more likely to be enrolled in facial recognition technology systems.
The thought of biases being a part of the perceived “scientific and neutral” role of technology has caused a lot of discussion. The exact reason as to why facial recognition systems perform differently on people of color has yet to be identified, however, there are several different reasons that could be potentially resulting in this. For starters, it could be that the individuals who are designing, programming and bringing these technological devices to life are overwhelmingly white and male. Those that get to be “in the room where it happens” represent a small, segmented, and privileged portion of the population. Therefore, it there is a potential that the algorithms developed for this kind of technology reflect the biases, as well as interests, of the populations who developed them. This is critical because the small population of people responsible for creating these algorithms are not representative of the larger population who is affected by the use of this technology.
Contributing Factors
Another reason as to why racial biases are present in facial recognition technology could be a result of the data that is used to teach or train this technology. Facial recognition systems are "trained" using data sets of faces, and often time the data sets skew towards white, and particularly male, faces. As a result, these algorithms are being taught how to effectively identify the faces of white males, and not so much the faces of anyone else. In attempt to remedy this potential causation, IBM has recently released a data set called Diversity in Faces, which is aimed at creating more diverse and representative data sets with which to train these types of technology. Diversity in Faces includes 1 million different images of human faces that include descriptive tags such as face symmetry, forehead height and nose length. This data set is "the first of it's kind available to the global research community" that "seeks to advance the study of fairness and accuracy in facial recognition technology."
While creating more diverse, accurate and representative data sets, or trying to diversify the people creating algorithms are both potential remedies to reducing the racial biases that are present in facial recognition technology, they are not perfect solutions. Creating dangerously accurate facial recognition systems still poses concerns and potential threats for communities of color. Just because a facial recognition technology works the same on everyone does not mean it works the same for everyone. In a society where people of color are policed and surveillanced at disproportionately higher rates than others, highly accurate facial recognition technology could still be used to survey and police these populations even more heavily. However, when facial recognition technology is so inaccurate that it is identifying Black people as "gorillas", it becomes even more unclear what the best solution is to remedy the current impact of these racial biases in technology.
While we are still figuring out how racial biases are manifested within facial recognition technology, as well as how best to eliminate them, it is clear we still have a lot of research to do in this area. There is still much work to be done in order to fully understand the scope of the potential harm of facial recognition technology on communities of color, as well as consider the implications of the solutions presented. While acknowledging the issue and attempting to instill diversity are important first steps, they are still first steps and we have a long ways to go. As Meredith Whittaker of the AI Now Institute said, "If the AI Industry wants to change the world then it needs to get its own house in order first."
READ MORE: 5 Ways to Minimize Expert User Bias, What to Know About Global Usability, Inclusivity and UXR Part 1: Revising Survey Screeners, Don't Turn a Blind Eye to Website Accessibility
Comments
Add Comment