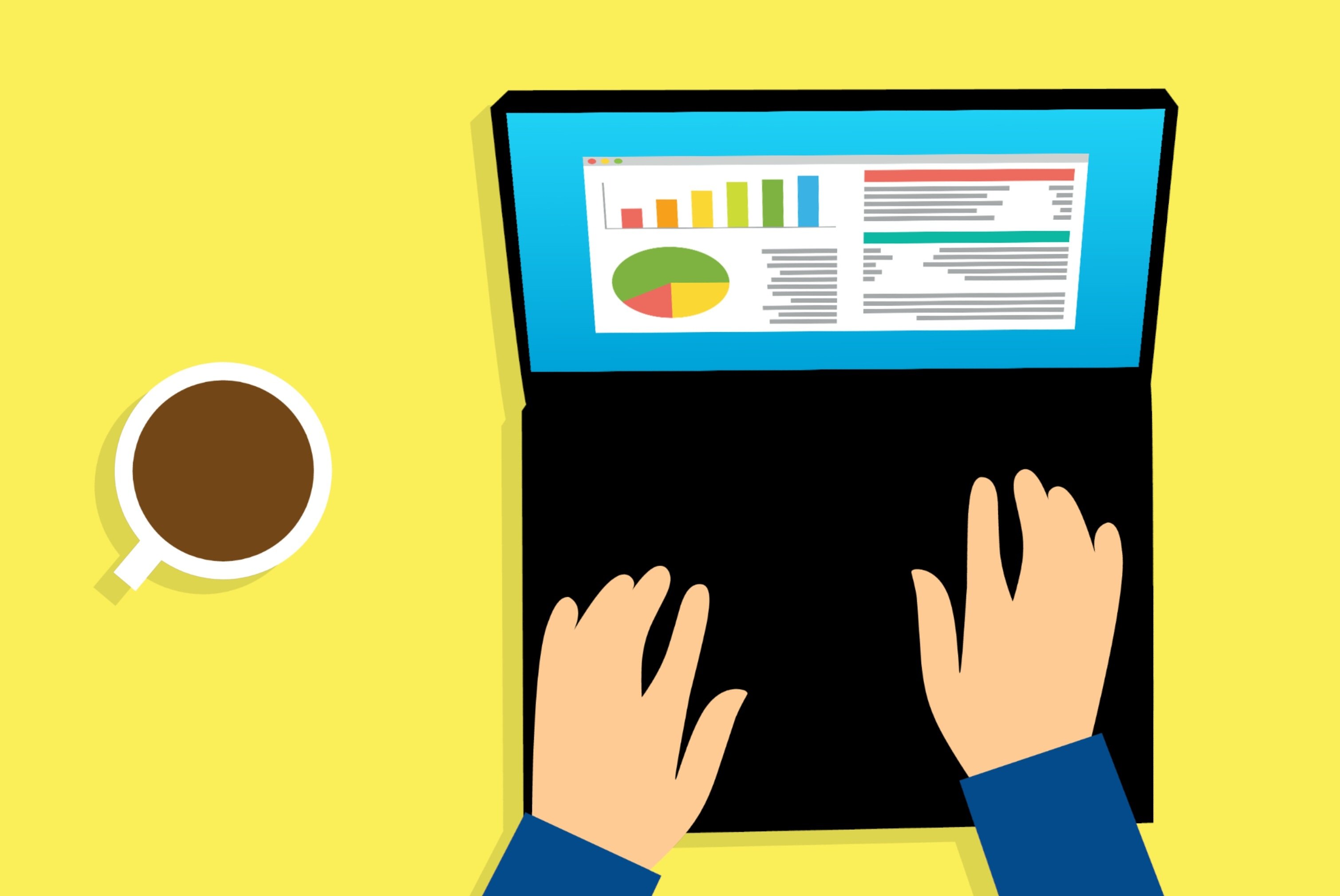
The ultimate benefactor of the current trend of focusing on customer experience (CX) is the customer.
What has created this trend towards CX? Competition. It’s become increasingly difficult for brands and products to differentiate themselves, so it’s smart to rely on data to guide decisions.
Generally speaking, data will allow you to make the best decisions from a risk vs. reward perspective, however, the most basic principle for customer satisfaction and a positive customer experience is often overlooked: Care for the customer at every level of the organization. For example, one of the hardest industries in which to differentiate and still be profitable, is the airline industry. Customer experience is one of the most meaningful differentiators between one carrier and the other. The pilot who says goodbye to every passenger as they deplane; the smiling flight attendant; or the attentive customer service representative. Customer care makes a brand likable.
The idea of “care” sounds simple, right? Research can help you understand where, when and how a customer feels “cared for.” The pandemic - and the world's regulatory response to it - forced us all to change our behaviors, and for companies to pivot to a more “digital first” customer experience. Today, over two years later, the world has changed and customers find it is inexcusable for brands not to be acquainted with their “new” behaviors and motivations, and to be able to provide a positive digital experience.
In the same way that the most basic principle for customer satisfaction can sometimes be elusive, when using summary measure variables to create insights from research findings, the basics are frequently overlooked as well.
The use of Mean, Median and Mode for different types of datasets
Today, there are a multitude of tools and information available to CX researchers about data analytics, however, the basics are not always considered. One of these basic principles that is frequently forgotten is deciding when to use summary measure variables such as a mean, median or mode.
When analyzing interval data where you know the exact difference between each quantity or variable - for example, age, income, or a measurement of distance - and there is order, any of the following summary measure variables can be used: mean, median or mode.
When analyzing ordinal data - a categorical, statistical data type where the variables have natural, ordered categories and the distances between the categories are not known - we can obtain mode and median, but not mean. We cannot measure the mean of ordinal qualitative data because it essentially means - no pun intended! - nothing, since we cannot quantify the exact size of the difference between variables. For example: What is the difference between “Strongly Disagree” and “Disagree?” The only exception is Likert scales that are built out of several Likert-scored items which are combined into a single composite score, and can be analyzed as interval data. There are many other non-parametric methods to analyze ordinal data like ordinal regressions, frequency distributions, top-two box, contingency tables, etc.
Finally, when analyzing nominal (relating to or consisting of names) data - purely descriptive data that cannot be hierarchized, ordered or ranked - we can only obtain the mode, since obtaining the median would require data that can be ordered, as in ordinal data. Frequency distribution tables or graphs are commonly used for this type of data, and will give you the mode at a glance.
When analyzing even richer datasets, like those produced by qualitative studies, a first pass to categorize the data might be required. A popular method to do this is theme analysis, where you tag data (observations, quotes, notes, etc.) with overarching themes or codes. Afterwards, these themes can be treated as nominal data.
Keeping these basics in mind when analyzing data from your UX and CX research is important because the data often inform high level product and organizational decisions.
How to make product and organizational decisions based on UX research data is a very complex topic, and it usually starts by running one or several quantitative studies to better understand the breadth of the issues you’ve identified through the qualitative, exploratory research and then engaging stakeholders across a broader array of business domains. There are numerous frameworks that help guide these conversations with stakeholders, which commonly take the form of workshops. When making these decisions, it is always important to map any changes to the overarching, high level customer journey to understand potential downstream effects. At Key Lime Interactive, we use JourneyTrack.io to help catalyze these conversations and workshops with stakeholders, to provide the big picture of the customer journey and to help prioritize and operationalize the proposed optimizations to the customer journey.
Comments
Add Comment