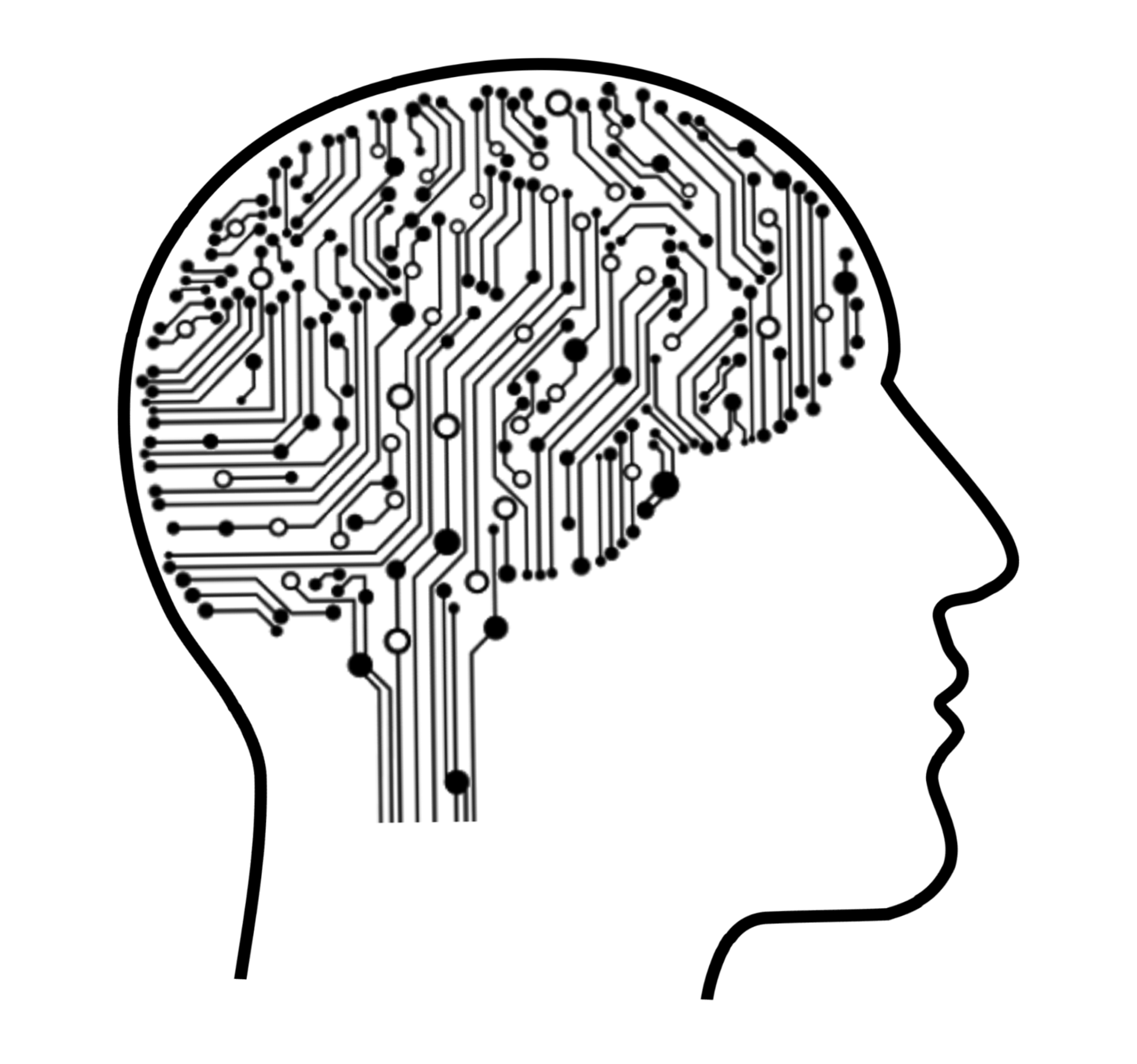
Machine Learning (ML) and Artificial Intelligence (AI) are two words that are being used a lot right now in the emerging technology world, and will probably only continued to be used more and more. Often time these words are used interchangeably, or used together, which causes their distinct meanings to become fuzzy and unclear. Machine Learning and Artificial Intelligence, while can be used together, are ultimately two distinct concepts. This article explores the difference between the two terms and why this difference is important to remember.
"Where machine learning is reactive, artificial learning is proactive." - Jeff Catlin
What is Artificial Intelligence (AI)?
So what exactly is Artificial Intelligence (AI)? Artificial Intelligence is a term that is thrown around all the time and making its way into everyday conversations. But just to make sure we are all on the same page here, AI refers to the concept and the development of computer systems that are able to perform different tasks such as visual perception, speech recognition or decision making- things that we feel normally require human intelligence. AI is a fancy-sounding way of referring to machine’s carrying out tasks that we could consider “smart” or tasks that would require some kind of intelligence to complete, like translating between two different languages.
What is Machine Learning?
On the other hand, Machine Learning (ML) is often used interchangeably with the term Artificial Intelligence because of the fact that it can be seen as an application or an extension of AI. It takes the current concept of artificial intelligence as a means of giving machines access to data and then letting them learn from that data themselves. This means that ML has the ability to automatically learn new information and improve itself from experiences without having to be programmed in a certain way since the machine will be able to teach itself new forms of information. Since ML has the ability to take data and “learn” from it, ML would be capable of doing things such as listening to pieces of music to decide which one best fits the mood, or reading a text and determining whether the person writing it is complaining or praising. This doesn't mean that ML is as simple as building something and having it automatically learn things; the design and input are still essential factors in making ML work in an effective way. ML requires the machine to be taking in new data to, therefore, make new decisions based on the new data; thus the machine “learning” or “teaching” itself.
The Difference Between Machine Learning and Artificial Intelligence
This is the critical point where ML and AI begin to differ and why it’s so important to recognize the distinction between the two. The machines are not sentient (yet) and need to be told when it gets something wrong, or given new data in order to figure out what the correct answer is. If new data or feedback is not given to the machine, it will not organically “learn” from its mistakes and adjust accordingly. As a result, ML doesn’t really do “feedback”; it needs to be told explicitly how to fix a problem. AI, on the other hand, operates off a feedback loop. Rather than having to input new data, AI is able to seek out new sources of data and rebuild itself based on those feedbacks, unlike ML.
This is what makes the difference between AI and ML so important to remember, especially for designers and coders. In order for ML to work and be effective, someone needs to know how to code and how to input data into the machine in a way that it can read and understand. AI, on the other hand, has to be designed in a way that is constantly seeking out new inputs or forms of feedback and making sure it is able to incorporate that moving forward. As technology continues to develop, change, innovate and move forward, we can be sure to hear the terms artificial intelligence and machine learning more and more.
READ MORE: The Role of UX in AI, The State of AI by Industry, Accessibility in UX, Customer Journey Map: AI Edition, What You Should Know About ZeroUI
Comments
Add Comment